If there’s an industry that needs AI to serve as an inflection point in its trajectory, it is drug development. Long before OpenAI opened the floodgates of the AI push, drug manufacturers had been exploring the potential of AI and machine learning to make drug discovery more efficient and cost-effective.
Developing a new drug typically costs between $1 billion and $ 2.3 billion and takes 6 to 12 years, depending on the trial complexity and regulatory hurdles. These high costs and lengthy timelines are pushing the industry to adopt AI-driven solutions to speed up development and cut inefficiencies.
This AI shift is already visible at an industry level. Lantern Pharma, for instance, used its AI platform, RADR, to bring three drugs into clinical trials in about three years and at a cost of under $3.5 million each. The typical time it takes is four to seven years.
“Three years is an unheard-of timeframe in cancer, and we’ve done that three times.”, Panna Sharma, chief executive of Lantern Pharma.
Since 2015, AI-driven biotech firms and pharmaceutical partners have introduced 75 molecules into clinical trials, with 67 still active as of 2023.
Over the past decade, this number has grown exponentially, with an annual compound growth rate exceeding 60%. This rapid expansion suggests that AI’s influence has a significant impact on clinical trials.
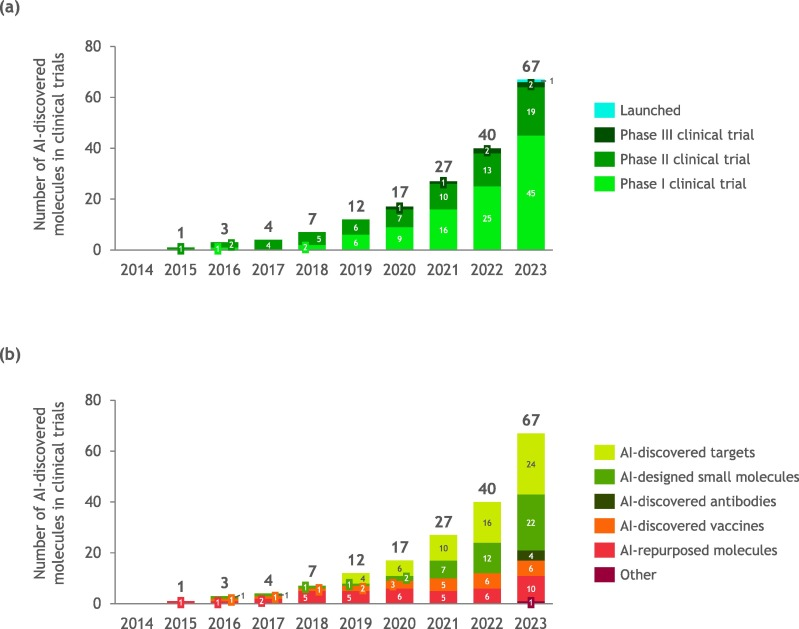
The bottleneck in drug discovery isn’t just cost and time
The actual bottlenecks lie in scientific complexity and clinical trial attrition. Despite advancements in tools, many diseases remain poorly understood, making accurate identification of drug targets a major challenge.
As AstraZeneca notes:
“Target identification lies at the heart of modern drug discovery. On paper, the process sounds simple—find a biological target that plays a role in disease, then find a therapeutic that interacts with it—yet this belies the complexity of the task.”
Clinical trials also remain a significant hurdle. 90% of drug candidates fail, primarily due to inefficacy, safety concerns, and inadequate pharmacokinetic profiles. Additionally, lack of efficacy results in over 50% of Phase III failures. This highlights the need for more accurate early-stage predictive models.
A Surge in Partnerships for AI-based Drug Development
Many prominent pharmaceutical companies, such as Sanofi, Janssen (a subsidiary of Johnson & Johnson), and AstraZeneca, are working to mitigate these challenges by partnering with AI-based drug discovery startups. This trend has become increasingly prevalent in recent years. Below are a few examples of big pharma companies partnering with AI-based biotech firms.
Sanofi Partners with Atomwise’s AI Technology to Accelerate Drug Discovery with 3 Trillion Compounds
Atomwise and Sanofi have entered into a strategic research collaboration. Sanofi will utilize Atomwise’s AI-powered AtomNet platform to discover up to five drug targets computationally.
The collaboration aims to improve drug discovery, particularly for challenging or poorly characterized targets. Atomwise’s platform utilizes deep learning for structure-based drug design. It enables rapid searches of its proprietary library, which contains over 3 trillion synthesizable compounds. This technology enhances the ability to identify and optimize compounds for complex biological targets, making the drug discovery process more efficient and rational.
Valo Health and Novo Nordisk Partner to Accelerate Cardiovascular Drug Discovery with AI-Powered Platform
Valo Health and Novo Nordisk have partnered to develop new treatments for cardiometabolic diseases using Valo’s Opal Computational Platform. This platform integrates real-world patient data, AI-driven small molecule discovery, and Biowire human tissue modeling.
The partnership aims to overcome the limitations of traditional drug discovery methods by enhancing the efficiency of target identification, validation, and drug development through the use of AI and human-centric data, thereby accelerating the development of novel therapeutics.
Novo Nordisk will license three preclinical cardiovascular drug programs from Valo and may collaborate on up to 11 more. Valo will receive $60 million upfront, with the potential for up to $2.7 billion in milestone payments and royalties.
Merck KGaA Leverages Quris-AI to Predict Drug Toxicity and Enhance Safety
Quris-AI has extended its collaboration with Merck KGaA, Darmstadt, Germany, following a successful preclinical study that demonstrated its ability to predict drug toxicity. Merck will now leverage the Quris-AI platform’s ability to identify liver toxicity risks when selecting drug candidates.
This platform outperforms traditional in vitro and in vivo methods by more accurately predicting drug safety, reducing reliance on animal testing, and cutting costs and time in drug development. Merck KGaA may secure an exclusive license to a specific disease domain depending on the success of further collaboration.
This collaboration addresses the limitations of traditional animal models and the complexity of predicting drug safety and toxicity in drug discovery. Improving the accuracy and efficiency of safety predictions could potentially reduce late-stage failures and the need for animal testing, thereby accelerating the drug development process.
Bristol Myers Squibb Partners with AI Proteins to Develop Therapeutic Mini-Proteins
Bristol Myers Squibb collaborated with AI Proteins, a Massachusetts-based biotech company, on a deal valued at up to $400 million. This partnership utilizes AI Proteins’ artificial intelligence-driven platform to discover and develop therapeutic mini-proteins targeting two undisclosed yet specific areas.
AI Proteins specializes in creating mini-proteins through computational protein design. These proteins are noted for their small size and high affinity. These characteristics offer advantages over traditional antibody therapies, including improved tissue penetration and faster clearance, which could enhance both efficacy and safety. The agreement includes an upfront payment, options for exclusive worldwide licenses on the developed mini-proteins, and additional target options.
While large pharma companies have made significant strides, smaller AI-driven biotech startups are pushing the boundaries of drug discovery with novel applications of AI. These startups are offering specialized platforms that provide agile and cost-effective solutions to streamline discovery processes. One such startup is Biomatter.
Exploring AI Startups Driving Innovation in Protein Engineering and Drug Discovery: Biomatter
Biomatter is a synthetic biology startup focused on developing novel proteins for applications in health and sustainable manufacturing.
Its Intelligent Architecture™ Platform is designed to overcome the limitations of traditional enzyme engineering methods by enhancing design accuracy and reducing development time.
The platform integrates generative AI with principles of physics, creating a unified digital system that leverages the strengths of both technologies.
It facilitates advancements in protein design by combining data-driven AI with physical modeling. It enables the precise modification of existing proteins and the creation of new proteins from scratch, leading to substantial improvements in protein function and properties.
The platform optimizes multiple parameters simultaneously to achieve design accuracy and speed. The engineered enzymes enable customized treatments that enhance the effectiveness of enzyme replacement therapies, potentially leading to new therapies for various medical conditions. It has multiple application segments:
- Protein Design: Enables broad design capabilities, allowing the creation of proteins with novel chemistry and functions.
- Protein Optimization: Rapidly improves biochemical and biophysical properties to fine-tune proteins for specific applications.
- Protein Discovery: Efficiently explores existing protein data to identify proteins with desired functions or properties.
- Generative Design: This approach utilizes tools such as ProteinGAN and AsiteDesign, which employ generative AI to expand the protein sequence space, thereby creating unique, functional proteins.
Biomatter has secured €6.5 million in seed funding, co-led by Inventure and UVC Partners.
Biomatter’s breakthrough in 2019, which created the first fully functional enzymes using generative AI, was published in Nature Machine Intelligence.
With a growing customer base that includes Thermo Fisher Scientific, BASF, and Neogen, this funding will support Biomatter in expanding its platform’s capabilities and developing de novo enzymes.
Biomatter Solutions
1. Intelligent Architecture™ platform
The Intelligent Architecture™ platform is designed to help biotech companies streamline and improve their research processes by quickly analyzing vast amounts of data.
The platform enables researchers to organize complex biological data and apply intelligent algorithms to predict how molecules, genes, and proteins behave in various environments. By knowing how these molecules respond to various conditions, researchers can make more accurate predictions about how drugs or treatments will interact with the body or specific diseases.
This makes the whole process of drug discovery and development much more efficient.
It further helps optimize enzymes efficiently, even without previous experimental data.
According to a study, it improved the performance of enzymes such as β-glucosidase and flavin reductase by up to 8 times, making them more stable by increasing their melting temperatures by up to 30°C, and enhancing their lasting activity. The platform works quickly, generating up to 30 enzyme designs in about a week. This reduces the need for extensive experiments and shortens project timelines.
2. ProteinGAN
ProteinGAN is an AI-powered tool that helps design new proteins capable of catalyzing chemical reactions. These reactions are crucial for various biological processes, such as digestion, energy production, and DNA replication. Traditional methods struggle with this process due to the complex relationship between protein sequences and their functions.
ProteinGAN uses a “self-attention” technique within a generative adversarial network (GAN). This enables ProteinGAN to learn from the natural diversity of protein sequences. It can then generate new ones that are likely to function well. Exploring the wide range of possible amino-acid combinations generates proteins with natural-like properties.
For example, ProteinGAN was tested with malate dehydrogenase (MDH), an enzyme. Of the 55 protein sequences it generated, 13 (or 24%) showed solubility and catalytic activity in lab tests. This included one sequence with 106 amino acid changes. This indicates that ProteinGAN can rapidly generate diverse and functional proteins within natural biological constraints.
This tool enables researchers to predict and design proteins with specific functions. It accelerates the discovery of new therapeutic proteins and enzymes. It also enhances the likelihood of identifying successful drug candidates.
3. AsiteDesign
Biomatter introduced a new method called AsiteDesign for enzyme engineering. This approach redesigns key parts of proteins, specifically catalytic residues, to alter or enhance their activity.
AsiteDesign utilizes a Monte Carlo technique and the pyRosetta framework to explore various shapes for protein active sites, which are essential for making enzymes more practical for industrial use.
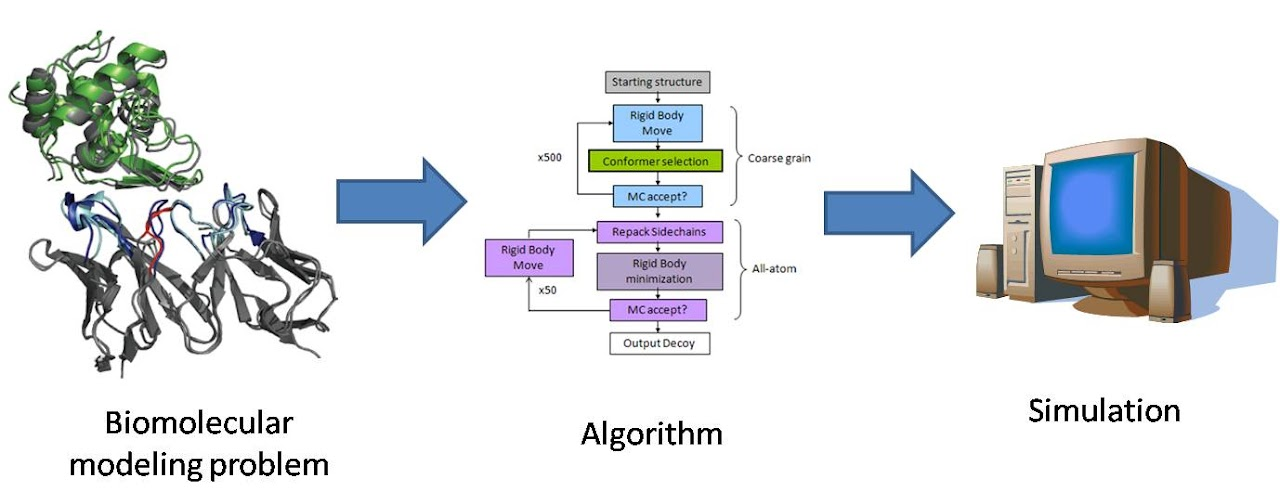
In a test, AsiteDesign was applied to an enzyme from Pseudomonas fluorescens, successfully improving its activity and selectivity. It helped the enzyme break down a large substrate more effectively. It also altered its selectivity, demonstrating the method’s ability to fine-tune enzyme functions.
This technique is especially valuable in drug discovery. When combined with Generative AI, AsiteDesign becomes part of Biomatter’s Intelligent Architecture™ platform. This platform can optimize enzyme functions for the development of new drugs.
Find out more startups to partner with and expedite your drug development. Reach out to us:
Path Forward
AI has great potential to improve drug discovery, but it also presents challenges, particularly in terms of data management. AI models require large, high-quality datasets; however, issues such as data bias, inconsistency, and relevance can impact their performance. When data is too limited or scattered, it becomes challenging to organize and interpret for critical research.
Furthermore, the complexity of biological systems renders it challenging for AI models to predict outcomes accurately. Biological interactions are often influenced by many factors, which can make predictions less reliable.
However, these challenges are being addressed, and AI’s role in drug discovery is set to expand further. With collaborations between big pharma and AI-driven biotech startups, such as Atomwise and Biomatter, reshaping the industry, the question remains: How can you leverage AI in your drug development pipeline? Get in touch to find AI drug development startups.